Exploring Deep Learning Algorithms: Types, Purposes and Real-Life Examples
- SCHEMOX
- Aug 13, 2023
- 5 min read
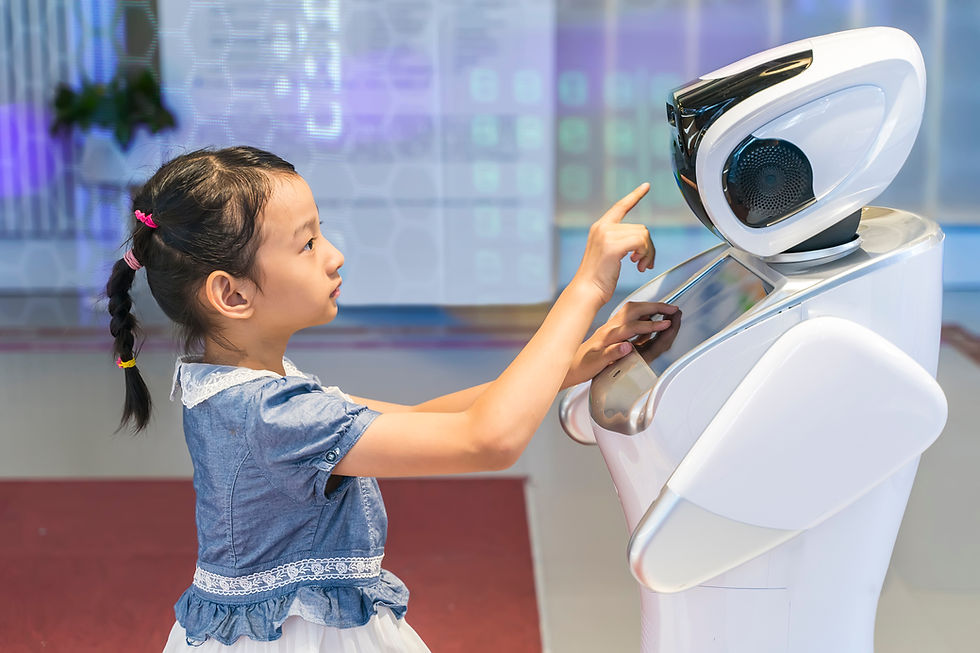
Table of contents
• Introduction
• Types of Deep Learning Algorithms
• Purposes of Deep Learning
• Real-Life Examples of Deep Learning
• Challenges Faced by Deep Learning Algorithms
• Future of Deep Learning
• Conclusion
Introduction
Let's talk about Deep Learning. Not to be confused with shallow learning; that's like only reading the headlines. Deep Learning is a subset of machine learning that deals with algorithms inspired by the structure and function of the brain, called artificial neural networks. In simpler terms, it's technology that can learn and make decisions like humans.
The concept of Deep Learning started way back in the 1940s when researchers designed the first artificial neuron, called the McCulloch-Pitts Model. The neural network has since come a long way, with advancements in hardware and software capabilities, powering the Fourth Industrial Revolution.
In today's world, Deep Learning has taken on a vital role in business and society, enabling real-world applications like facial and speech recognition, self-driving cars, and recommendation systems. It's the tech that can transform industries, revolutionize lives, and open doors to grander visions in the future.
Types of Deep Learning Algorithms
Deep learning is a subfield of machine learning that utilizes neural networks to model and solve complex tasks. There are various types of deep learning algorithms that have specific use-cases. Convolutional Neural Networks (CNNs) are excellent at tasks related to image recognition, object detection and classification. They work by extracting features from the image and comparing them to a set of predefined features. Recurrent Neural Networks (RNNs) are suited for sequential data, such as text or speech. They are used for tasks such as text classification, translation, and speech analysis.
Deep Belief Networks (DBNs), on the other hand, are specialized for unsupervised learning tasks, such as anomaly detection and clustering. They consist of multiple layers of hidden nodes that extract features from the input data and produce a compressed representation of the original data. Generative Adversarial Networks (GANs) are a type of deep learning algorithm that can generate new data by learning from existing data. They consist of two networks: a generator network that creates new data samples, and a discriminator network that tries to distinguish between real and fake data samples.
The final type of deep learning algorithm is Autoencoder Networks, which are used to learn a compressed representation of the input data. They are commonly used for tasks such as data denoising, reducing dimensionality and image reconstruction. Understanding the types of deep learning algorithms is essential for determining the right approach for a given task.
Purposes of Deep Learning
As Deep Learning continues to make strides, its application in various fields is becoming more apparent. One of the main purposes of Deep Learning is image recognition. By training neural networks using vast amounts of data, machines can now detect objects, identify faces, and even describe an entire image.
Another crucial purpose of Deep Learning is Natural Language Processing (NLP). Machines can now translate one language into another, summarize text, and even generate coherent sentences. With Speech-to-Text Processing, machines can convert human speech into text in real-time, enabling applications like voice assistants to be more efficient.
Lastly, Predictive Analytics is also a key purpose of Deep Learning. With machine learning algorithms, businesses can now identify patterns in data, make accurate predictions, and even automate decision-making processes.
Undoubtedly, the application of Deep Learning in various fields has revolutionized businesses and many aspects of everyday life. From self-driving cars to virtual assistants and recommendation systems, the potential for Deep Learning is limitless. However, some of the challenges it faces are overfitting, underfitting, and evaluating performance. Nevertheless, Deep Learning is poised to transform our lives in more ways than we can imagine.
Real-Life Examples of Deep Learning
Deep learning is increasingly becoming ubiquitous across several industries with a range of applications that would have been considered impossible just a few years ago. Here's a sneak peek at some groundbreaking examples of deep learning that have been making waves in real life.
First up, self-driving cars. The automotive industry has been one of the earliest adopters of deep learning technologies. By using machine learning, computer vision, and neural networks, self-driving cars can perceive and interpret their surroundings, identify obstacles, and make decisions in real-time. While not yet perfect, the strides made in the development of self-driving cars have been astounding.
Another industry that is benefitting from deep learning is virtual assistants. Devices such as Alexa and Siri have been integrated with deep learning algorithms, making them capable of understanding and responding to natural language queries with near-human accuracy. As a result, virtual assistants have been making our daily lives a whole lot easier and more convenient.
Facial recognition software is another example where deep learning has been gaining ground. The technology can identify and verify an individual's identity with impressive accuracy, improving security and convenience across several industries, including health care, finance, and law enforcement.
Voice recognition software also uses a variant of deep learning called Recurrent Neural Networks (RNNs) and Convolutional Neural Networks (CNNs) to convert speech to text. Advances in deep learning have resulted in the development of voice assistants that understand everyday language better than ever and respond faster than ever.
Lastly, recommendation systems like those used by Amazon, Netflix, and YouTube use deep learning to analyze data about users' behaviour, interests, and habits, and make predictions on what content to recommend next. This feature has helped businesses improve customer satisfaction, engagement, and sales.
Overall, deep learning is transforming industries across the board, bringing innovation, improved efficiency, and greater automation to the way we do things.
Challenges Faced by Deep Learning Algorithms
When it comes to Deep Learning, it's not all smooth sailing. Even with the incredible capabilities of deep learning algorithms, there are still several challenges that must be dealt with along the way. One of the biggest problems is overfitting, which is when the model becomes too good at fitting the training data and starts to perform poorly on real-world data. Another issue is underfitting, which is the opposite of overfitting and happens when the model is too generic or simplistic to properly learn from the data.
One more significant challenge is the vanishing gradient, where the gradient shrinks during backpropagation, making it harder for earlier layers to learn. Finally, evaluating performance can be difficult, since accuracy isn't always the best metric to use. Regardless of these challenges, Deep Learning algorithms are still an excellent way to improve business processes and improve the overall quality of life. Understanding these challenges will only help to improve the effectiveness of Deep Learning algorithms in the future.
Future of Deep Learning
The future of deep learning looks incredibly bright with endless opportunities in various fields. In healthcare, deep learning can help in detecting diseases promptly and accurately, leading to better treatment outcomes. Education can leverage deep learning to personalize learning and allow for more accessible and engaging methods of delivering education. Agriculture can boost productivity by analyzing massive amounts of data to improve farming techniques. Enhanced automation and efficiency can streamline business workflows, improve predictability and reliability, and ultimately increase profits. The potential for deep learning is unlimited, and as time progresses, we are likely to see new and exciting use cases in every industry. Who knows, maybe deep learning might soon take over the world! (Just kidding, or am I?)
AI's Impact on Humanity
0%Benifits Humanity
0%A Threat
0%Depends on its Usage
Conclusion
To sum it up, Deep Learning algorithms are transforming the world we live in and have endless potential applications. From self-driving cars to virtual assistants, the algorithms are becoming more complex. However, as with any technology, challenges like Overfitting and Underfitting exist. As their potential grows, so does the future scope, including applications in Healthcare and Agriculture. We are witnessing the next industrial revolution, thanks to Deep Learning algorithms.
Comments